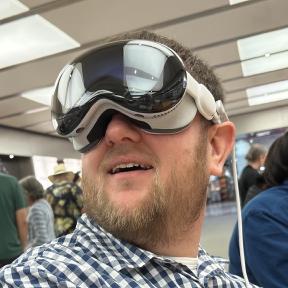
Kevin Conley
Software Engineer
A little about me:
I’ve been lucky to have worked on the following projects:
🚛 dev experience for autonomous truck software developers at Stack AV
💳 managed dev environments and tooling for
Square Point of Sale devices
🤖 HIL test automation for
Intel Optane solid state drive firmware
👓 graphics firmware for the
Intel Vaunt smart glasses
⌚️ app/UI firmware for Pebble smart watches
I also enjoy drinking cold brew, solving puzzles, playing video games, and birdwatching.